
- This event has passed.
Symposium on Information Interaction and Learning
April 25 @ 2:00 pm - 5:00 pm
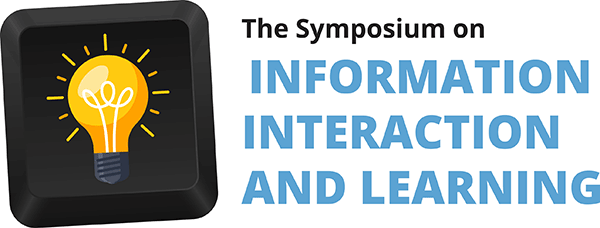
The School of Information and Library Science (SILS) is pleased to host the second annual Symposium on Information Interaction and Learning. The aim of the Symposium is to disseminate research related to interactive information retrieval, the learning sciences, and education. The Symposium is open to all and will feature six lectures.
- Jeffrey Greene and Matthew Bernacki (UNC School of Education) will present research on detecting struggling undergraduate students using the digital trace data they generate through their normal course engagement.
- Anita Crescenzi (UNC SDSS & SILS) will present research on the relationship between time pressure, information search, decision making, and the use of novel search tools.
- Kelsey Urgo (University of San Francisco) will present research on the effects of goal setting on learning during search.
- Hamed Zamani (University of Massachusetts Amherst) will present research on personalizing large language models (LLMs) to help users learn about topics and find answers to their questions.
- Negin Rahimi (University of Massachusetts Amherst) will present research on interpretable search algorithms.
- James Allan (University of Massachusetts Amherst) will present research on algorithms for finding prerequisite concepts to help searchers learn.
The second annual Symposium on Information Interaction and Learning is being organized by Dr. Jaime Arguello and Dr. Rob Capra (UNC SILS). The Symposium is sponsored by the U.S. National Science Foundation through Project Athena, a collaborative research grant between UNC SILS and UMass aimed at developing tools to help people learn while searching for information. For more information on Project Athena, visit https://ils.unc.edu/~jarguell/athena/ and https://ciir.cs.umass.edu/research/athena.
Details
Thursday, April 25 from 2–5 p.m.
UNC Student Union, Room 3411
Lectures
A Learning Theory and Analytics Paradigm for Prediction and Support of Student Learning
The transition to higher education can be an abrupt shock for students who have not developed the knowledge, skills, and dispositions necessary to do college-level work. Many of these students struggle in their first semester, threatening their short-term retention and long-term success. Educators want to help these students, but typically learn of their students’ difficulties only after they fail a major exam, which can be too late to provide sufficient support. In this presentation, we will detail our research on identifying and supporting these students. Using only the digital trace data students generate via their normal course engagement, our team has developed theory-based, empirically-demonstrated learning analytics models that after only two weeks of class, can predict with 80% accuracy which students will struggle to pass a course. These models allow us to deliver targeted, science of learning to learn interventions to these students before their first exam, to help them succeed. We will present evidence that completion of these interventions leads to significant improvements in exam performance, increasing the likelihood of short- and long-term success.
About the Presenters
Jeffrey A. Greene is the McMichael Distinguished Term Professor of Educational Psychology and the Learning Sciences in the Learning Sciences and Psychological Studies program at the University of North Carolina at Chapel Hill. He is also a Distinguished International Professor at the Hector Research Institute, University of Tübingen (appointed from June 1, 2023 through December 31, 2025). His main area of scholarship involves how to help people become effective, efficient, and critical users and creators of information in technology contexts. He has published over 100 peer-reviewed journal articles, conference proceedings, books, and book chapters on self-regulated learning, epistemic cognition, and online learning, among other topics. He is a Fellow of the American Psychological Association and the American Educational Research Association.
Matthew L. Bernacki is an Associate Professor of Learning Sciences and Psychological Studies and Donald & Justeen Tarbet Distinguished Scholar at the University of North Carolina at Chapel Hill. He is also a Distinguished International Professor at the Hector Research Institute, University of Tübingen (appointed from June 1, 2023 through December 31, 2025) and a K-CLUB faculty member in the Brain and Motivation Research Institute at Korea University (appointed from January 1, 2024 to December 31, 2025 for an initial term). He has published over 65 peer-reviewed journal articles, conference proceedings, books, and book chapters on self-regulated learning, motivation, metacognition, and learning with technology including those that personalize and adapt instruction. He leads the Center for Learning Analytics in the School of Education, and works with educators and designers to use the data students produce when they learn to study their self-regulated learning process.
Time-pressured Information-seeking
Information-seeking and learning take place in a variety of conditions – including time-pressured situations. Time pressure is known to have an impact on the process and outcomes of information search as well as the use of novel information system features. In this talk, I will describe a series of studies that have found a relationship between time pressure, information search and decision-making, and the use (or non-use) of novel features of information search tools. I will tie this to upcoming exploratory research that will include when and why people choose to use search versus generative AI tools to resolve their information needs.
About the Presenter
Anita Crescenzi is an Assistant Professor in the School of Data Science and Society and the School of Information and Library Science at the University of North Carolina at Chapel Hill. In her research, she focuses on understanding how people use information-seeking systems in support of their broader goals, as well as design and evaluate novel information-seeking systems and interaction features to better support learning, problem-solving, and decision-making. More recently, her research has expanded to include human-centered data science, including data search and reuse. She has her BS in Secondary Education from the University of Illinois at Urbana-Champaign and her MSIS and PhD from the School of Information and Library Science at UNC. She has also worked as a middle school teacher and a user researcher and user experience designer in industry and medical library settings.
Exploring the Influence of Subgoals on Learning During Search
Over the past decades, human search needs have evolved. Search tasks have moved from simple fact-finding to involving cognitive processes such as analyzing, evaluating, and creating (processes that are central to complex human learning). However, modern search systems still lack support for these complex human tasks. In this talk, I will discuss a pair of studies that investigated the influence of goal-setting on learning during search and the self-regulated learning processes that seem to be important to learning during search.
About the Presenter
Kelsey Urgo is an Assistant Professor at the University of San Francisco. She earned her PhD from SILS at the University of North Carolina at Chapel Hill. Her research involves information retrieval and human-computer interaction. The intersection of these fields, interactive information retrieval, is concerned with both search environment development and human search behaviors and cognition. Her current work specifically is in search-as-learning and involves the development of search environments to support complex human learning online.
Personalizing Large Language Models
Many users these days rely on Large Language Models (LLMs) to learn about topics and find the answer to their questions. In this talk, I will discuss models and evaluation methodologies for generating personalized outputs, depending on the user’s preferences, history, or background knowledge. In more detail, I will first introduce the Language Model Personalization (LaMP) benchmark — a large-scale benchmark for studying personalization for text classification and generation using LLMs. I will later draw connections between LLM personalization and retrieval-enhanced machine learning (REML) and introduce retrieval-augmented approaches for personalizing large language models.
About the Presenter
Hamed Zamani is an Assistant Professor in the Manning College of Information and Computer Sciences at the University of Massachusetts Amherst (UMass), where he also serves as the Associate Director of the Center for Intelligent Information Retrieval (CIIR), one of the top academic research labs in Information Retrieval worldwide. Prior to UMass, he was a Researcher at Microsoft. His research focuses on designing and evaluating statistical and machine learning models with applications to (interactive) information access systems and retrieval-enhanced AI systems. His work has led to over 90 refereed publications in the field, in addition to a number of open-source research tools. His research has been recognized by a CAREER Award from NSF, Early Career Excellence in Research and Community Engagement awards from ACM SIGIR, multiple research awards from Adobe, Amazon, Google, and Microsoft, and multiple paper awards from SIGIR 2023, SIGIR 2022, CIKM 2020, and ICTIR 2019.
Where do I begin? Finding concept prerequisites in a collection of documents
Although an incredible amount of search, particularly on the web, looks to find relatively straightforward answers to questions (when does Target close tonight?), sometimes we want to make sense of lots of data so that we can learn about a large and complex topic (how do LLMs work?). We explore one version of this challenge, supporting a searcher by creating a “reading path” through concepts in a collection of topically-related documents – for example, indicating that transformer models and important background reading for LLMs. We describe approaches to this problem and some weaknesses they exhibit, particularly with the datasets used for training and evaluation. After covering our own approach to constructing training data, we detail our approaches to identifying key concepts and their prerequisite relationships. We conclude with early results and next steps.
About the Presenter
James Allan is a Professor in the Manning College of Information and Computer Sciences at the University of Massachusetts Amherst. He is currently the Associate Dean for Research and Engagement for the college and directs the Center for Intelligent Information Retrieval (CIIR). His research focuses on ways to process, organize, retrieve, and browse unstructured text information, and he is particularly interested in using language tools to support critical thinking by readers. His other major research activities look at ways to recognize contention and misinformation in text, to support effective interactive search techniques, and to build and evaluate more effective search models on large collections of within- and cross-language text.
Toward Interpretable Models for Obtaining Diverse Answers
Existing information access systems, including those that employ large language models (LLMs), face challenges in appropriately providing users with diverse, unbiased, and well-explained responses to explore the answer space. In this talk, I will present a summary of our work on interpretable information retrieval. I will provide a more detailed description of our efforts to enhance interpretability of models for providing users with diverse and unbiased results. Through enhanced interpretability, we have achieved comparable or even higher effectiveness.
About the Presenter
Negin Rahimi is an Assistant Professor in UMass Amherst’s Manning College of Information and Computer Sciences. Her research is on information retrieval with a focus on interpretable information access. Her research is supported by Google Research Scholar, Adobe, and NSF awards.
Made Possible by a Grant from the National Science Foundation
Grant Number: IIS-2106334
Grant URL: https://www.nsf.gov/awardsearch/showAward?AWD_ID=2106334